Unlocking the potential of set-based design with machine learning
Unlock the power of set-based design in automotive engineering with Secondmind. Leverage advanced machine learning to streamline complexity and boost data efficiency, so engineers can discover innovative designs faster and more effectively.
Modern cars now feature 100 million lines of code to drive, yet a Boeing 787 Dreamliner only requires 14 million lines of code to fly [1]. Today’s vehicles are more complex than ever, synergizing mechanical, electrical and software together with the latest ADAS, connectivity and autonomous technologies to deliver the performance and reliability drivers’ demand.
This is making the job of automotive engineers extremely challenging. ‘Historically, vehicles were mechanical machines, then electrification came along, and now they have evolved into fully software-defined vehicles,’ highlights Morgan Jenkins, Chief Product Officer at Secondmind. ‘The integration of all these disciplines increases the pressure on automotive engineers.’
‘Now, engineers must develop a solution that suits a myriad of competing requirements, in shorter timeframes and with smaller budgets,’ adds Jenkins. ‘One of the best strategies to achieve this is to utilize set-based design to understand the feasible design space much earlier in the development cycle. This facilitates shifting left by moving V model processes earlier in the timeline.’
The traditional point-based design approach
The typical approach to developing a product follows the point-based design methodology, which commits to a single solution early in the design process, before thoroughly considering all the downstream implications.This solution is then re-worked through numerous iterations to improve performance.
However, point-based design often leads to incorrect design decisions which are discovered too late, resulting in any adjustments becoming costly as teams rush to meet deadlines. This leads to shortcuts and compromises, affecting the final quality and functionality of the product.
Point-based design is even more ineffective when it comes to developing products with higher complexity. With more parameters to consider, trying to identify the optimum solution without fully understanding the potential design space means the final product is more likely to miss design objectives.
- How generative AI can solve complex automotive engineering problems
‘As complexity increases, the natural tendency of engineers is to simulate more,’ explains Jenkins. ‘However, the fidelity required to model this higher complexity makes simulations computationally expensive and time intensive. It’s a familiar frustration when engineers are having to make compromises because of point-based design and on top of that, simulation takes so long that the design has evolved, making the results irrelevant.’
‘Relying on simulation to deliver answers quick enough is no longer sustainable for such complex applications,’ continues Jenkins. ‘Yet, the automotive industry is very accepting that simulation takes time, and point-based design means we rarely arrive at a final product that meets all the design requirements in an optimal way. But why should we accept this? That is the question Secondmind asks because we believe there is another way.’
Advantages of set-based design
An alternative approach is set-based design, where multiple design options are investigated simultaneously. As tests are completed and assumptions are validated, designs are gradually eliminated over time. This ensures the final design is a result of proactively selecting options that match the desired requirements, rather than committing to one solution early and correcting it to meet specification.
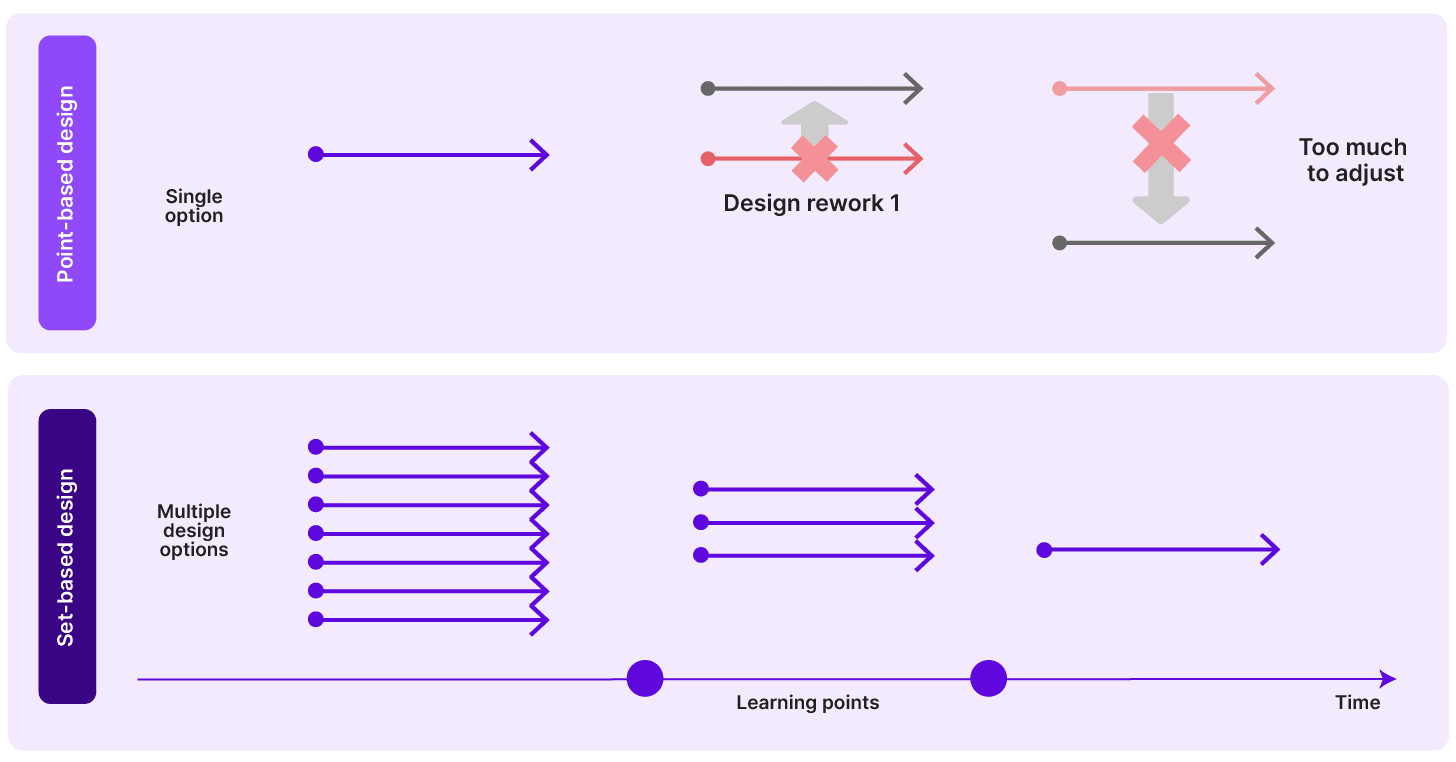
Set-based design explores multiple concepts simultaneously, ensuring the final design aligns with requirements
‘Set-based design predicts feasible options within a multi-dimensional design space, rather than taking a single point solution and predicting its performance,’ says Jenkins. ‘These feasible design candidates are then given to different departments, who can innovate with confidence. When these developments are integrated back together, there is a much higher probability that everything will work because everyone has been developing from the same robust, feasible options that were originally defined by set-based design.’
However, managing the vast amount of data associated with set-based design can be a barrier to its adoption. Each potential design needs to be conceptualized, prototyped, tested and analyzed to establish its performance against requirements. With set-based design exploring thousands of initial design options, this can lead to an exponential increase in data, which current tools struggle to cope with. So how can organizations capitalize on the benefits of set-based design, without drowning in data and computational costs?
The Secondmind solution
The answer lies in leveraging Artificial Intelligence (AI), which is exactly what Secondmind for System Designachieves. This proprietary software utilizes innovative AI strategies to deliver data-efficient set-based designpractices, enabling engineers to explore all design options quickly and effectively.
Secondmind for System Design works by first connecting to existing simulation tools and then running Secondmind Active Learning in the cloud. This intelligently maps the design requirements with performance targets and uses probabilistic models to predict feasibility for given inputs. In this way, feasible options are quickly identified within a multi-dimensional design space and then visualized to help engineers focus on the points that offer the greatest potential.
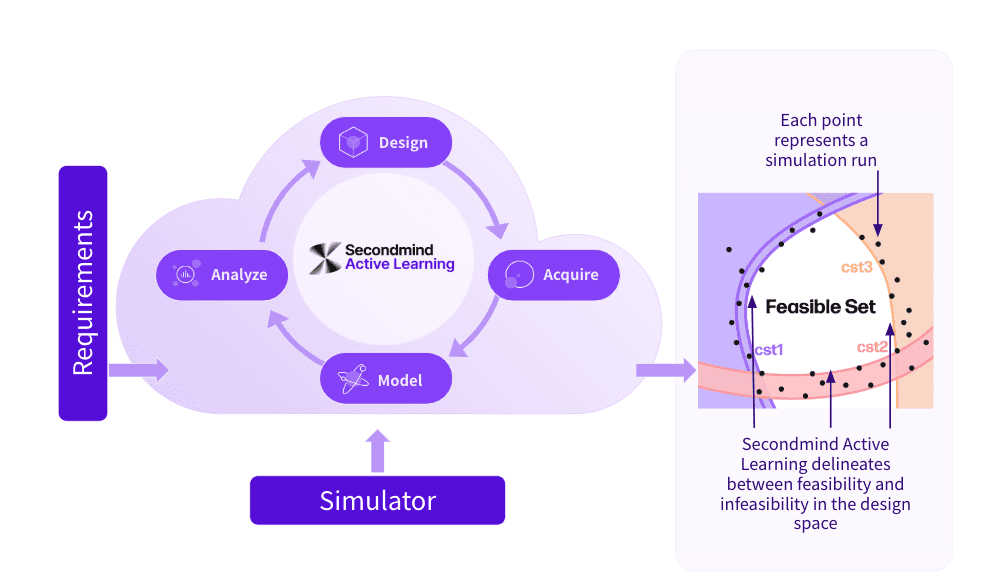
Secondmind for System Design plugs into existing tools and predicts the feasible design space framed by boundaries of constraints
‘The options near the fringes of feasibility are potentially more uncertain but innovative,’ explains Jenkins. ‘Before, engineers did not have enough confidence to explore these areas, and so would choose more conservative solutions. However, Secondmind helps engineers understand the level of uncertainty in these areas, which they can then use to analyse trade-offs. This, along with the transparency within the model, gives engineers the confidence they need to explore regions that they were not comfortable exploring in the past.’
Data-efficient AI automotive design
One of the major benefits of Secondmind for System Design is data-efficiency. Secondmind’s algorithms have been developed to explore the design space in an intelligent and automated way, only requesting data when necessary and therefore minimizing the number of required simulations. This reduces the amount of data required by up to 80% and requires just 20% of the simulations needed for traditional approaches, saving companies a significant amount of time and money.
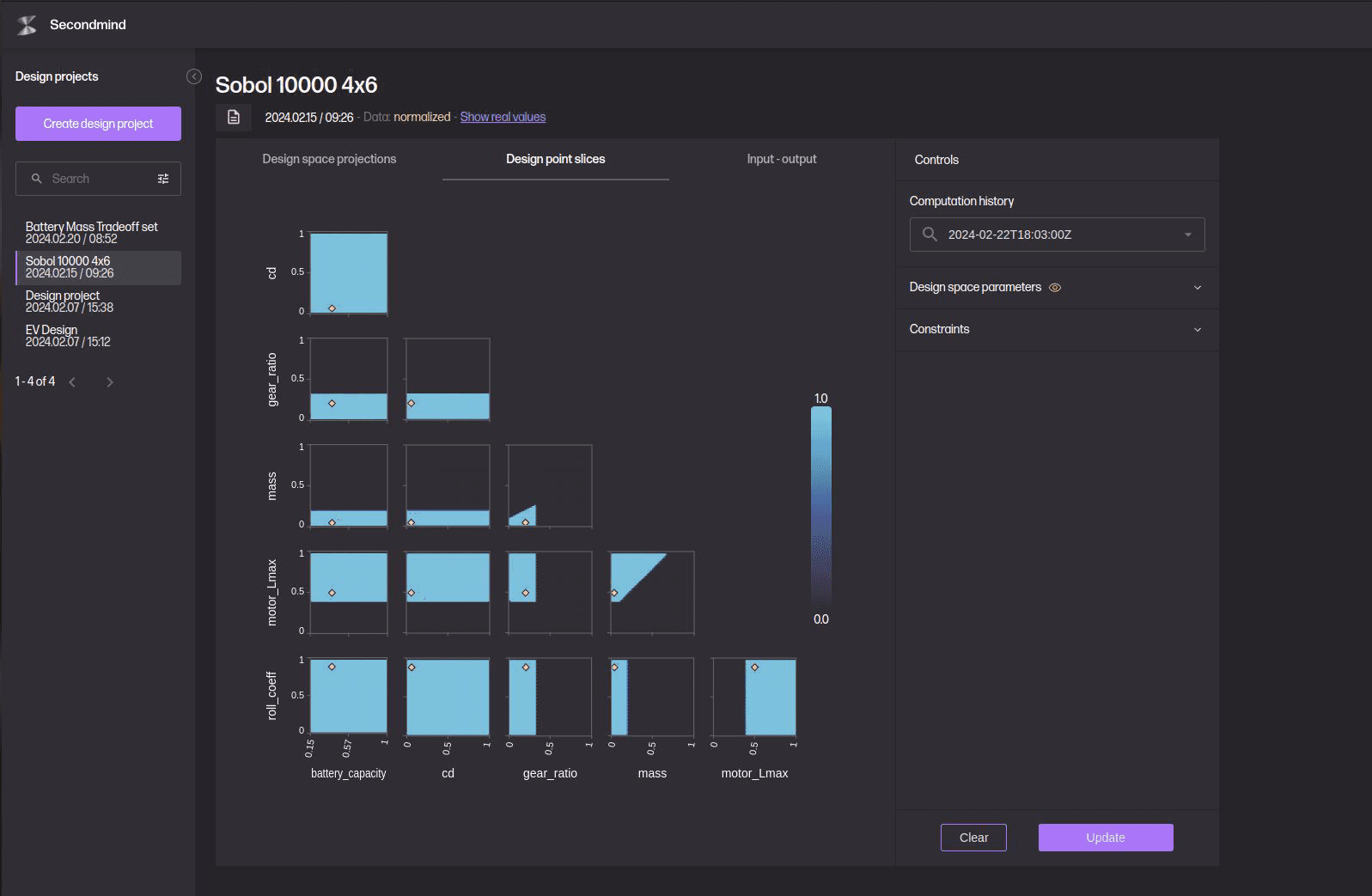
Design space projections for a 6x6 multi-dimensional design space with feasible designs highlighted in blue and constraints represented by the black areas
‘Overall, the complexity of modern automotive engineering problems is now so high that set-based design really is the only approach to developing innovative, but effective solutions,’ concludes Jenkins. ‘However, simulating this higher number of potential options takes too long, generates too much data and costs too much.’
‘We offer a new way of exploring the design space, identifying feasible but innovative options quickly and efficiently, whilst integrating with current workflows and tools,’ adds Jenkins. ‘The real power of Secondmind for System Design comes when it is used during the conceptual phase of the V model. Its influence then cascades through the rest of the processes, feeding all departments and supply chains, helping them improve the fidelity of the product whilst always remaining feasible.’
Find out how our machine learning algorithms work in our latest white paper here
References
[1] M.M., 2021. When software writes software [Online]. Porsche Newsroom.