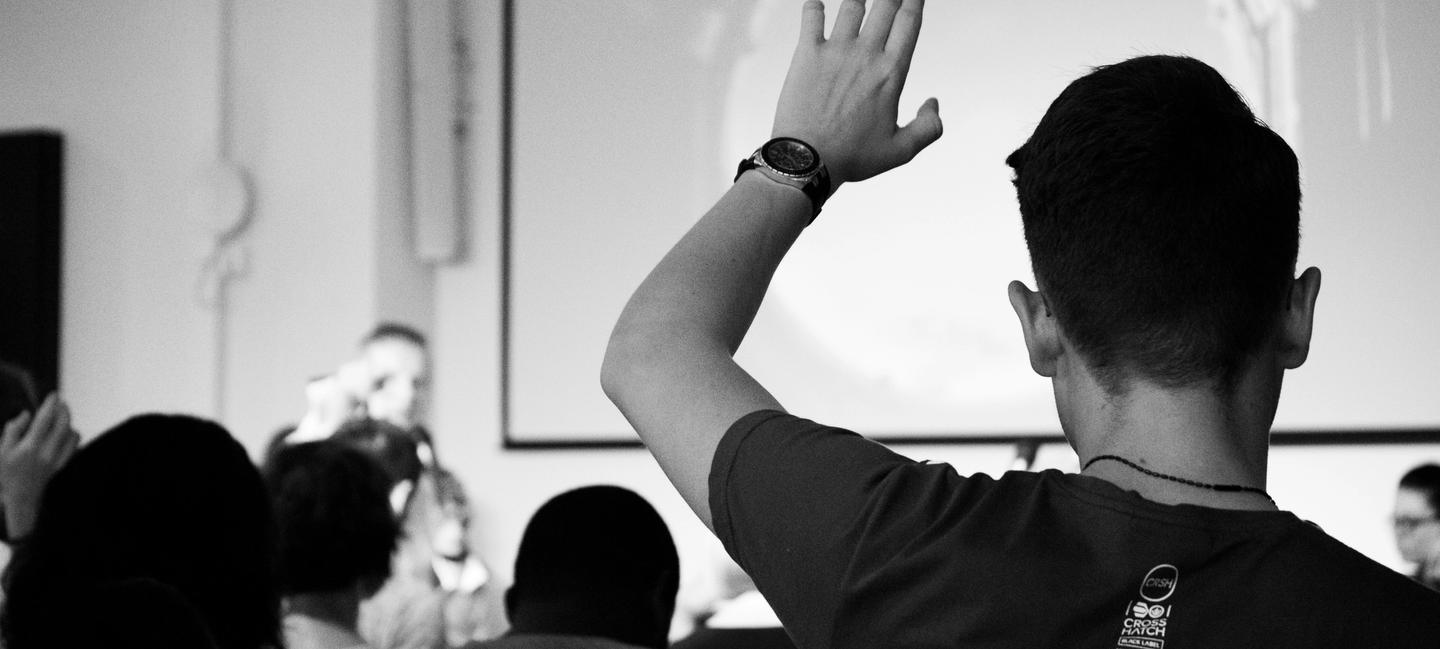
Seminar: Ítalo Gomes Gonçalves - Universidade Federal do Pampa
Variational Gaussian processes for spatial modeling: the geoML project
Abstract
The Earth is capable of producing very complex phenomena. The mining and oil industries are constantly challenged to model complex geological bodies, the concentration and distribution of valuable metals, and so forth. Data is usually scarce and low-dimensional, and good uncertainty estimates are critical. This scenario makes the Gaussian process (GP) the ideal model. This work aims to leverage the recent advancements in the variational GP in order to deal with asymmetric data distributions, non-Gaussian likelihoods, multivariate modeling, non-stationarity, and other situations. The latest development is an analytical deep GP model that employs a convolutional kernel to propagate uncertainty through the layers. This allows the setup of an irregular network, modeling the dependencies between variables in a way that best suits a given problem's geological premise.
Notes
- Reference: Learning spatial patterns with variational Gaussian processes: Regression
- Personal website can be found here .