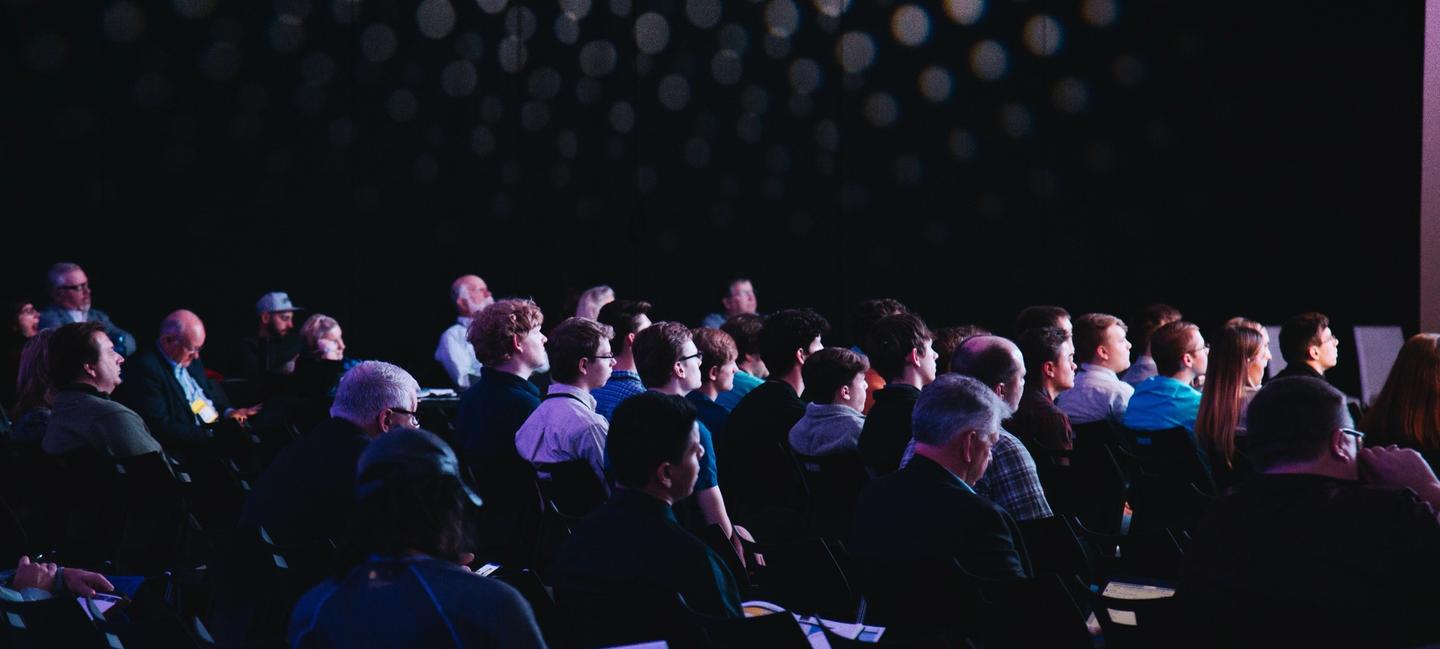
Seminar: José Miguel Hernández Lobato - University of Cambridge
Probabilistic Methods for Increased Robustness in Machine Learning
Abstract
Most machine learning methods are brittle and their performance degrades catastrophically when the input data distribution changes. In this talk, I will describe two probabilistic approaches to address this problem. First, to obtain methods that degrade gracefully, I will focus on scaling accurate approximate Bayesian inference to large neural networks. I will show that it is enough to perform inference over a small subset of the model weights to obtain accurate predictive posteriors. The resulting method, called subnetwork inference, achieves very significant improvements when making predictions under distribution shift. Second, to avoid performance degradation in specific cases, I will describe invariant Causal Representation Learning (iCaRL), an approach that enables accurate out-of-distribution generalization when there is training data collected under different conditions (environments). iCaRL achieves generalization guarantees by assuming that the latent variables encoding the inputs follow a general exponential family distribution when conditioning on the outputs and the training environment. Experiments on both synthetic and real-world datasets show that iCaRL produces very significant improvements over existing baselines.
Notes
- Dr. José Miguel Hernández Lobato is a University Lecturer in Machine Learning at the Department of Engineering in the University of Cambridge, UK. His website can be found here .