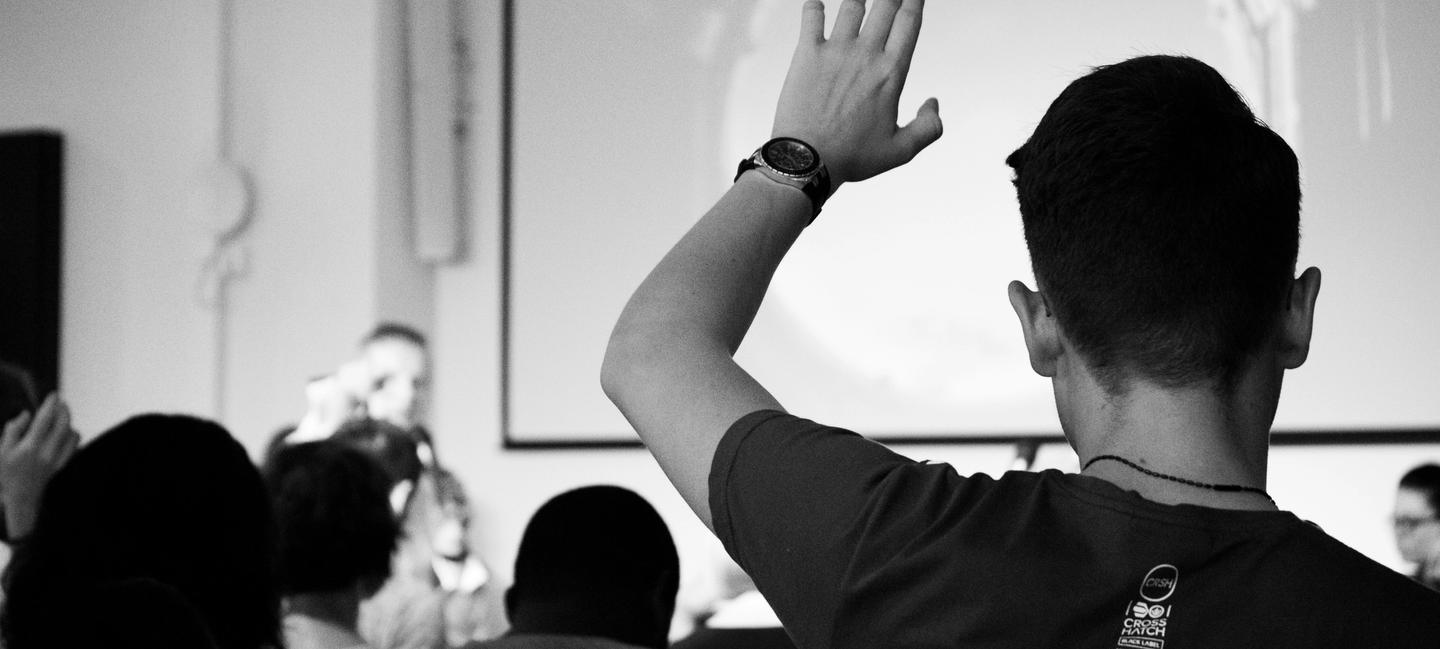
Seminar: Arno Solin - Aalto University
Stationary Activations for Uncertainty Calibration in Deep Learning
Abstract
We introduce a new family of non-linear neural network activation functions that mimic the properties induced by the widely-used Matérn family of kernels in Gaussian process (GP) models. This class spans a range of locally stationary models of various degrees of mean-square differentiability. We show an explicit link to the corresponding GP models in the case that the network consists of one infinitely wide hidden layer. In the limit of infinite smoothness the Matérn family results in the RBF kernel, and in this case we recover RBF activations. Matérn activation functions result in similar appealing properties to their counterparts in GP models, and we demonstrate that the local stationarity property together with limited mean-square differentiability shows both good performance and uncertainty calibration in Bayesian deep learning tasks. In particular, local stationarity helps calibrate out-of-distribution (OOD) uncertainty. We demonstrate these properties on classification and regression benchmarks and a radar emitter classification task.